The Role of Artificial Intelligence (AI) in Clinical Outcome Assessments (COAs)

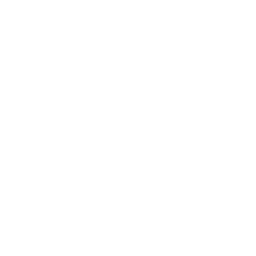
Abstract
Artificial Intelligence (AI) is the new frontier for content creation, reuse of language assets; saving time and effort in the curation of documentation. The widely adopted generative AI tools, such as ChatGPT have altered the landscape, in many instances transforming authors into editors. But can the same be said for all content? Can clinical documentation be treated like non-scientific documentation?
COAs in Clinical Studies
COAs are carefully, scientifically balanced, stress-tested Measurement tools used in patient data capture in a clinical trial. The 21st Century Cures Act (Cures Act, Public Law 114-225), defines COA as ‘‘(A) a measurement of a patient’s symptoms, overall mental state, or the effects of a disease or condition on how the patient functions; and (B) includes a patient-reported outcome.”
Capturing patients’ biometric data during a study is quantitative; however, capturing the patient experience i.e., their experience taking part in the study, with the study drug etc. is more qualitative, so item selection is not a simple exercise in creating a questionnaire for general population, but more measured and defined.
Can AI Differentiate the Subtle Complexities of Scientifically Defined Items?
Utilizing COAs1 as metrics to assess AI interventions is essential for gaining a deeper understanding of how these interventions impact a patient’s well-being, including improvement in symptoms and quality of life. While the main objective of AI interventions may be to enhance workflow efficiency and to aid clinicians in decision-making, it remains imperative to gauge whether such interventions yield positive outcomes, or at the very least, do not adversely affect the overall lived experiences of patients.
As outlined earlier, AI has significant potential in content creation, authoring, regulatory and literature review. These disciplines share one common thread: Volume.
AI has been described as a data hungry technology. In other terms, it requires large data sets to make predictions and find and actuate base models.
Challenges for COA in AI Systems
AI lends itself well to large datasets. It is these large datasets that inform and train the AI for best results. But not all aspects of a study are verbose. Many aspects are lean and draw from a limited word count. COAs or Measures are a set of scientifically defined questions that are crafted to deliver the patients experience of a study drug and that of the study itself; delivering qualitative2 data that cannot be gathered by biometric assessments per se.
COAs are notably short in length; it is almost impossible to confidently offer an average number of items in a questionnaire, but arbitrarily let’s say the average Measure is <40 individual items, it is easy to understand this dataset does not lend itself to AI instantly. The first consideration noted above is the small dataset size. The COA data collection is fragmented and might not be a true representation of the diverse target population desired2. This under representation of certain ethnic groups informs the learning of the AI system and therefore creates a scenario where the AI system not only lacks large datasets but also makes assumptions that may not be accurate.
Lastly, these AI systems are trained and measured against a clinician’s perceptions rather than the patient outcome data. This in turn gives prioritization of clinician observations over the actual patient experience. The flip side is where we rely on the AI perception and the uncritical acceptance of the clinician as the source of truth and accuracy.
As AI becomes more pervasive, not just in the clinical space but in real life diverse populations, there is a risk of inadvertent and unintentional bias3. Many of these AI algorithms can create a blind opaque scenario4 where clinicians are not able to clearly define the origins and integrity of said data.
Indeed, language translations of these Measures go through a meticulous process5 to ensure that no bias is introduced into the Measure. This demanding language translation process, in combination with a cognitive debriefing component with patients, reduces the risk of introducing bias into the process. Would AI be able to divine those intricacies?
Considerations
As mentioned above, due to small datasets used in COA, collecting COAs as standard across the breadth of medical interaction would be very useful. This contributes both to the betterment of patient care and health outcomes, while also enhancing the capabilities of algorithms that support care across multiple conditions, continually incorporating the patient experience."
Particular consideration should be given to not just the measure to be used in the study, but to the actual acquisition of patient study data.
AI is a significant benefit, not just to the clinical world but to content creation broadly. In clinical studies, it is a useful tool in content creation and curation, where large data sets are available. There is considerable work being carried out on leveraging AI in Protocol design6. The research market is primed for colossal growth due to the increased volume of clinical knowledge. That which is being observed can be deemed “the hockey stick effect” of increasing digitizing data and the ability to leverage that data in a meaningful way. The Pharma industry is forecast to spend $4.5B by 2030.7 Where smaller datasets are prevalent, leveraging those larger portions of the study documentation may be used to inform the betterment of the patient.
References
1. Center for Drug Evaluation and Research (no date) Clinical outcome assessment (COA): FAQs, U.S. Food and Drug Administration. Available at: https://www.fda.gov/about-fda/clinical-outcome-assessment-coa-frequently-asked-questions (Accessed: 14 November 2023).
2. Commissioner, O. of the (no date) Clinical Trial Diversity, U.S. Food and Drug Administration. Available at: https://www.fda.gov/consumers/minority-health-and-health-equity/clinical-trial-diversity (Accessed: 14 November 2023).
3. Char, D. S., Shah, N. H., & Magnus, D. (2018). Implementing Machine Learning in Health Care - Addressing Ethical Challenges. The New England journal of medicine, 378(11), 981–983. https://doi.org/10.1056/NEJMp1714229
4. Martín Noguerol, T., Paulano-Godino, F., Martín-Valdivia, M. T., Menias, C. O., & Luna, A. (2019). Strengths, Weaknesses, Opportunities, and Threats Analysis of Artificial Intelligence and Machine Learning Applications in Radiology. Journal of the American College of Radiology : JACR, 16(9 Pt B), 1239–1247. https://doi.org/10.1016/j.jacr.2019.05.047
5. Principles of good practice for the translation and cultural adaptation process for patient-reported outcomes (PRO) measures (no date) ISPOR. Available at: https://www.ispor.org/heor-resources/good-practices/article/principles-of-good-practice-for-the-translation-and-cultural-adaptation-process-for-patient-reported-outcomes-(pro)-measures (Accessed: 14 November 2023).
6. Li, V. O. K., Lam, J. C. K., Han, Y., Cheung, L. Y. L., Downey, J., Kaistha, T., & Gozes, I. (2021). Editorial: Designing a Protocol Adopting an Artificial Intelligence (AI)-Driven Approach for Early Diagnosis of Late-Onset Alzheimer's Disease. Journal of molecular neuroscience : MN, 71(7), 1329–1337. https://doi.org/10.1007/s12031-021-01865-z
7. How Artificial Intelligence Can Improve Clinical Trials (2023) Healthcare Weekly. Available at: https://healthcareweekly.com/artificial-intelligence-clinical-trials/ (Accessed: 14 November 2023).